SemanticPOSS
The latest information on this dataset could be found on the website of POSS Lab, Peking University, Beijing: http://www.poss.pku.edu.cn/semanticposs.html
Overview
SemanticPOSS contains 2988 LiDAR sweeps with a large quantity of dynamic instances in a campus-based environment. The dataset was collected at Peking University via and used the same data format as SemanticKITTI . The dataset can be used for semantic segmentation task. And for the sake of convenience, this dataset uses the same data format and interface as SemanticKITTI.
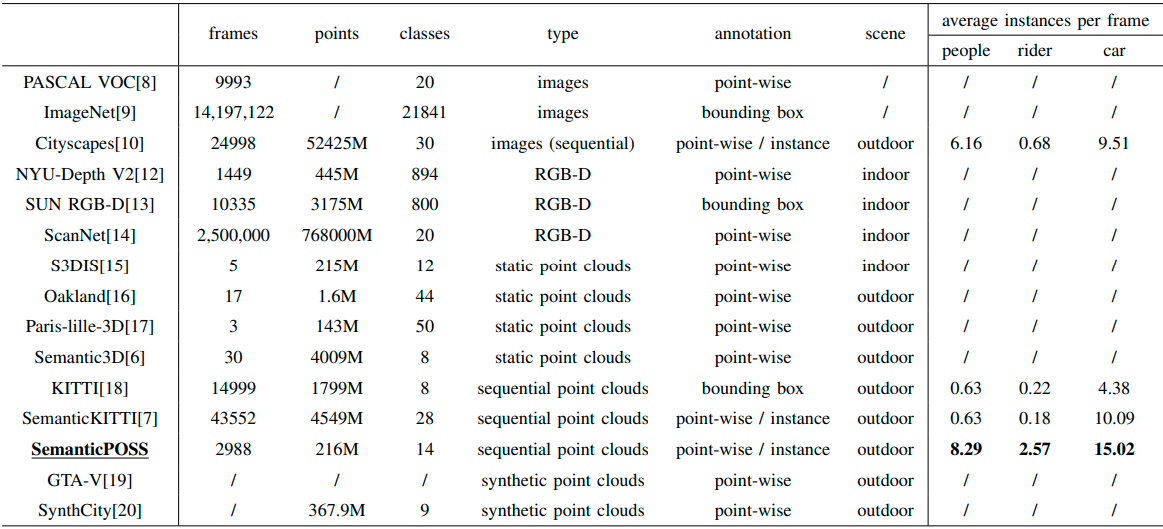
Classes and Data Format
The dataset contains 14 classes:
Class name | Class id |
---|---|
unlabeled | 0 |
people | 4, 5 |
rider | 6 |
car | 7 |
trunk | 8 |
plants | 9 |
traffic sign | 10,11,12 |
pole | 13 |
trashcan | 14 |
building | 15 |
cone/stone | 16 |
fence | 17 |
bike | 21 |
ground | 22 |
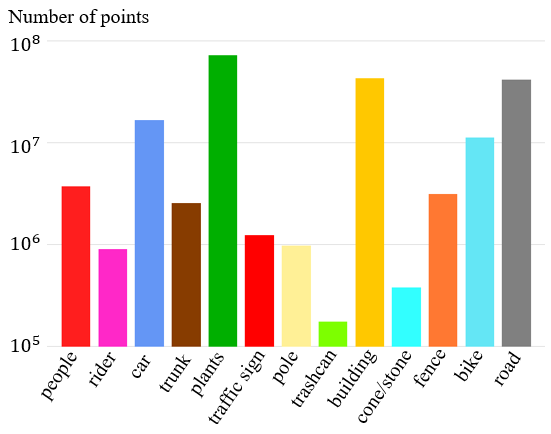
The folder structure and data format are the same as SemanticKITTI dataset. File XXXXXX.bin
in velodyne folder is the point cloud data. File XXXXXX.label
in the labels folder contains a label in binary format for each point. The label is a 32-bit unsigned integer for each point, where the lower 16 bits correspond to the label. The upper 16 bits encode the instance id, which is temporally consistent over the whole sequence. File XXXXXX.tag
in the tag folder is used for generating range image, which records the position of each point in range image.
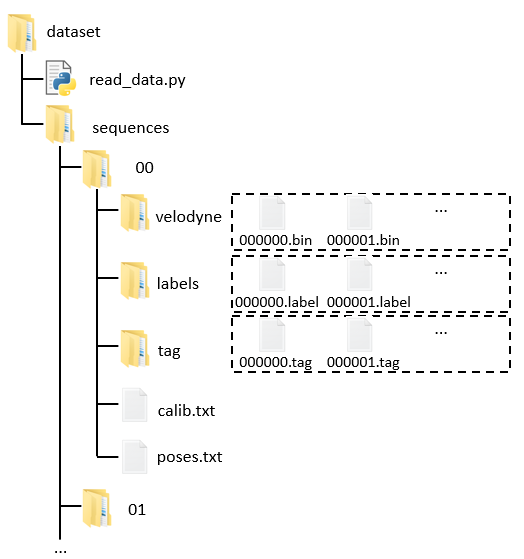
Citation
If you find our work useful, please consider citing it as
@INPROCEEDINGS{9304596, author={Y. {Pan} and B. {Gao} and J. {Mei} and S. {Geng} and C. {Li} and H. {Zhao}}, booktitle={2020 IEEE Intelligent Vehicles Symposium (IV)}, title={SemanticPOSS: A Point Cloud Dataset with Large Quantity of Dynamic Instances}, year={2020}, volume={}, number={}, pages={687-693}, doi={10.1109/IV47402.2020.9304596} }